This article first appeared in the 5th edition of The International Comparative Legal Guide to: Product Liability 2007; published by Global Legal Group Ltd, London (www.iclg.co.uk).
I. Introduction
Product liability refers to legal claims in which an injured party may recover some type of monetary compensation from the manufacturer or seller of a product. In the United States, the claims generally associated with product liability stem from claims of:
- negligence;
- strict liability;
- breach of warranty;
- and consumer protection.
Product liability litigation arises from a range of circumstances in which a specific product or product use comes under scrutiny. Manifestations of issues with a product include product failure, product misuse, and adverse side effects associated with an otherwise properly functioning and properly used product. A product liability claim tends to fall into one of three possible types: design defects, manufacturing defects, and failure to warn. Depending on the nature of the liability claim, different issues must be addressed. For example, product liability claims arising from some form of negligence (such as failure to warn) may require showing there is a duty owed to a party, that the duty was breached, and that the breach resulted in injury. In contrast, manufacturing defects often focus on "strict liability" in which the manufacturer is held liable for a defective product even if they are not directly implicated in the processes that led to the defect. An example of strict liability can be seen in the recent pet food recall in which a pet food maker in North America was held responsible even though they unknowingly purchased a contaminated ingredient from companies in China.
Whatever the nature of the liability at issue, analysis of product liability claims typically involves determining how the product was used, what customers’ expected of the product, what representations were made to customers about the product, and its failure rate. Such analysis is based on information that must be collected from product purchasers, users, or people exposed to the product as well as on the performance of the product. In addition, product liability actions often involve products that have been manufactured in the thousands or millions of units and consumers’ purchase, use, or exposure may reflect equally large numbers. The pet food contamination resulted in a recall of more then 60 million cans and pouches of food with untold numbers of pets exposed. Given the nature of the information required in these actions and the size of the population of products and consumers, sample surveys are an important tool. Sample surveys can address such things as how the product was purchased, the characteristics of the situation or environment in which the customer used the product, and any associated suffered damage. Further with the careful application of sampling techniques, data can be collected on smaller groups and the information can be extrapolated to the population of people or products.
In this chapter we review the uses of samples and surveys in product liability litigation, describe examples in which surveys played an important role, and discuss how surveys should be designed and implemented to produce reliable expert testimony. We then review the analytic framework that we use to quantify potential liability exposure in product liability cases in general; this framework can rely on estimated failure rates measured with a survey.
II. What is a Sample Survey?
We use the term sample surveys in this chapter to refer to a range of techniques in which a sample of elements from the relevant population is identified and a systematic procedure for asking questions, and sometimes measuring other conditions, is developed and followed. This is a broad definition and is intended to emphasise both the use of sampling techniques and the "systematic" process of asking questions.
Commonly, questionnaires may address opinion or expectation questions such as what were consumer expectations for the safety of a product. These types of survey questions focus on consumers or decision-makers state of mind or understanding of an issue. Labels applied to such surveys in the context of product liability litigation include opinion questionnaires, opinion surveys and consumer expectations surveys.
Also applicable are surveys that provide information on a range of other relevant issues associated with the purchase, use, and/or exposure to a product. These surveys may focus on collecting key facts about the purchase process such as whether the consumer recalls seeing specific advertising or whether they purchased the product in a particular store. Sample surveys used to measure key "factual" information about the use of the product have no particular label but are also often referred to as sample surveys.
Finally, there are sample surveys that combine asking questions and measuring physical conditions such that the survey responses of consumers and the actual conditions of a product can be linked. For example, in construction product defect cases it may be important to link specific physical conditions, such as water stains around a window, with information on how the homeowners use that window, in particular whether the window is always closed or whether it is left open from time to time allowing rain in. Thus, the term sample survey is used to identify a range of information collection processes in product liability cases.
III. Why Sample Surveys in Product Liability?
There are at least six reasons why the use of sample surveys may be found in product liability litigation.
- To determine the who and how of a product’s use . In many cases knowledge of who uses or has used the product and what conditions surround the use of the product may be at issue. In these cases survey and sampling procedures may be proposed as a method to advise the court on the nature and extent of a product’s distribution, use, or misuse as well as a product’s rate of failure or defect. In Perez v. John Deere Const. Equipment Co., Plaintiff Ernesto Perez alleged that the design of John Deere’s cotton picking machine was defective; Perez had been injured after becoming entangled with the head of the machine and argued that it was defective because there was no emergency shut off switch that he could have reached after becoming entangled. The Defendant utilised survey data that estimated the average number of hours the product at issue was used per year to demonstrate that the number of accidents involving this machine was only a small portion of the total number of hours the machine was in use (Perez v. John Deere Const. Equip. Co., * WL 1529762 (Cal. Ct. App. 2005)). These product event rates, such as purchase, usage, and failure rates, measured using surveys, are key components in assessing liability and in forecasting damages. The collection of this type of information is not only important for cases that go to trial, but also for negotiations in settlements or in negotiations between carriers and those insured.
- To determine consumers’ reliance on and materiality of product . In cases where a key issue is the nature of the exchange of information about the product and its characteristics (such as warranties, representations, warnings, etc), surveys can measure key facts about the exchange. For example, surveys may serve to address issues of who received information, what they received, how they received it, and whether the information was relied upon and/or material to some decision. In a construction defect, pre-certification case involving a roofing product, plaintiffs claimed the manufacturer misrepresented the product’s future performance. Surveys done by the defense showed that the majority of homeowners had purchased the homes from a developer, had no direct involvement in the purchase of the product and typically had not seen any warranties or marketing materials. Therefore, the manufacturer could not have misrepresented the product’s performance to the consumers as they did not receive any representations from the manufacturer.
- To apply the "Reasonable Consumer" test of consumer expectations. Under various legal theories of liability, a product or practice may be evaluated with a "consumer expectations" test or a "reasonable consumer" test. In such instances, surveys would be used to establish consumer expectations or what a reasonable consumer might expect of a product. In a case involving the lack of battery disconnects in 1999 Ford Mustang GT’s, Plaintiff Tunnel claimed breach of implied warranty by arguing that Ford failed to meet a reasonable consumer expectation regarding a battery disconnect device. In terms of establishing reasonable consumer expectations, Tunnel offered circumstantial evidence of industry awareness of the hazard and also offered direct evidence in the form of a consumer expectations survey. The court found in alleging an unreasonably dangerous design defect, direct and circumstantial evidence of reasonable consumer expectations of safety standards is admissible to show defectiveness (Tunnell v. Ford Motor Co., 330 F. Supp.2d 707 (W.D.Va. 2004)).
- To model "But-for" performance as a basis for damage claims . Sample surveys may also provide a basis for modeling the "but-for" conditions. In this type of use, a sample survey using hypothetical choice questions is designed and respondents are asked about their expectations, likely decisions, or the economic value of a product under specific alternative hypothetical conditions. These alternative conditions can represent the world minus the offending action. Consumers or business decisions under these alternative scenarios can then be used to make inferences about damages by comparing responses under current conditions to responses in the "but-for" world. Surveys to model "but-for" conditions are used, for example, in Cook v Rockwell International Corp. in which potential home buyers were asked to estimate the likelihood they would buy property a certain distance from a contaminated area and what discounts may be required to convince them to make a purchase if they otherwise would not (Slip Copy, (D.Colo. 2006)).
- To analyse the requirements for class certification . Many product liability claims seek some status as class action. In seeking class action status, surveys are used to address certain key issues related to the presence or absence of numerosity, typicality, and commonality of claims in a putative class. Surveys have been used both to support claims for class certification and to defend against them. For example, in a case claiming damages due to the alleged failure to properly disclose information about the content of a product, a survey was designed to assess both numerosity and commonality among a putative class of purchasers of the product.
In situations where product liability is at issue, surveys have been used both in the context of litigation and in analyses of product liability risk for financial disclosure, insurance related issues or corporate risk assessment. As such, sample surveys are a key tool for measuring inputs into a wide range of product liability forecasts (for a more detailed summary of product liability forecasting, (see Lucy Allen, Denise Martin, Simona Heumann, Paul Hinton, and Faten Sabry, "Forecasting Product Liability by Understanding the Driving Forces," International Comparative Legal Guide Series, Product Liability 2006, Chapter 7).
IV. Design and Implementation of Sample Surveys in Product Liability Cases
The careful design and implementation of a survey is critical to producing relevant and reliable results. Experts need to be prepared to prove their survey analysis meets objective standards of reliability to be admitted into evidence by the court. According to the Federal Judicial Center’s Manual for Complex Litigation, (See Federal Judicial Center, 1995. Manual for Complex Litigation, Third. Section 21.493, page 102) a well designed and implemented survey has the following features:
- the population was properly chosen and defined;
- the sample chosen was representative of that population;
- the questions were clear and not leading;
- the survey was conducted by qualified persons following proper interview procedures;
- the data gathered were accurately reported;
- the data were analysed in accordance with accepted statistical principles; and
- the process was conducted so as to ensure objectivity.
The first two features of a well designed and implemented survey, listed above, relate to the issues of population and sampling. The next two relate to questionnaire design and survey implementation and the final three issues relate to reporting and analysis of the survey results. We discuss each area in turn.
A. Population and sampling issues
In product liability cases the populations from which samples may be taken include: the entire population of products manufactured or distributed, the population of events involving the use of the product, and/or the population of people that use the product. Each is potentially large. Frequently the population may include thousands, tens of thousands and in some cases millions of products, events or people. How these populations are sampled for study is vital to whether sample survey results will provide reliable population estimates. The careful application of statistical sampling principles overcomes issues of bias or lack of generalisability. The keys to a reasonable sampling plan are:
- clearly defining the population of interest;
- applying sampling techniques that assure the sample is "representative" of the population; and
- choosing a sample size that will meet the required precision or confidence interval for the extrapolation.
1. Defining the population
First, it is critical that the population of products, people, or events is properly defined. One of the major challenges to whether a sample survey is probative is whether the correct population was studied. Defining the correct population usually requires a clear understanding of the legal claim and to whom it applies. For example, in a class action lawsuit over a roofing product, the class was defined as all persons who own or owned a home with the product installed during the years of the class. A sample was designed to destructively test a sample of the roofs and then use the results to provide an estimate for the entire class. However, at the time of trial, it was determined that the sample of destructively tested homes could only be extrapolated to the homes that had this product on the roof at the time the sample was drawn. This meant that the sample could not be used to extrapolate the rate of defect back to the population of roofs that had been previously replaced by the owners. Since the class included homes that were up to 25 years old, nearly one-third of the homes that had originally existed with this product no longer had the product on the roof. This ultimately reduced by nearly one-third the number of class members who could prove their case because the sample could not be used to generalise to all roofs that had had the product.
2. Ensuring the sample is representative
The next key is to assure that the method of sampling yields a "representative" sample of the population. Perhaps the most important tool for assuring representativeness is to use random sampling. Surprisingly, this is often ignored in product liability cases. Frequently, in product liability cases, the focus is on the product that has "failed." Thus, tests are done to establish what caused the failure and only "bad" product is actually tested. Alternatively, a few "bad" exemplars are tested and then a few exemplars that have not failed are tested. In either case, the generalisation of results from these procedures is questionable because there is no basis to determine the extent to which the samples tested are representative of the population. Any bias that is introduced by the haphazard sampling procedures may affect the accuracy of the population estimates.
Consider, for example, a construction defect case. An expert will be retained to inspect and perhaps destructively test units of the product. Based on that testing, the expert will estimate the frequency of the occurrence of defects for the entire population even though the expert has not inspected the entire population. Establishing that the defect rate in the sample is representative of the rate in the population is key to the experts’ opinion. If the procedures for selecting the sample can be shown to have been biased in any way, then the estimates of defect rates in the population will be unreliable. If, for example, the expert chose to test only products that could be easily attained, that were available at the time or that were available in a certain location (i.e. a convenience sample), then a question arises about the representativeness of these samples and the reliability of population estimates derived from the analysis. An example of this issue is seen in Dodson v. Ford Motor Co., in which a defense expert tested a sample of ignition switches. The court excluded the testimony because it believed the pool of switches was a biased sample mainly because the switches were provided to the expert by the defendant and because the evidence showed that the sample was not a random sample of such switches (Dodson v Ford Motor Co., *WL 2405868 (R.I.Super. 2006)).
While there are instances where simple selection of exemplars is useful, the most appropriate way to address sampling issues is through use of random sampling techniques. This means that sample surveys or measurements should be conducted on products or locations or people that are randomly chosen. Random sampling for the most part involves developing a list of the population elements (i.e., a list of the product that is at issue, or a list of the people that are affected) and then choosing a sample from this list using random numbers which are generated using a computer program. In many instances no complete list is available and procedures are used to develop a list that approximates the population. These procedures to develop a proxy list are often the focus of debate between experts with regard to their reasonableness in defining the population. Any bias associated with how the proxy list is created leads to debate over whether the correct population has been studied. For example, no list of everyone with a certain type of window may exist at either the manufacturer or the distributor. However, there may be a list of warranty registrations. In some instances the warranty registrations may be a sufficient proxy for the population and can be used for sampling. In other cases the experts may attempt to demonstrate that the people who fill out warranty cards are different than the people that do not, and therefore a sample based on warranty cards will be biased.
3. Determining the necessary sample size
The final key issue in sampling is sample size. There is no single right or wrong answer about how large a sample should be. What generally drives decisions about sample size is the cost to acquire the data and the level of precision required for the extrapolation. The precision of a sample is associated with what is called sampling variation. Basically, if you draw three random samples from the same population and compute the percent of respondents with a particular opinion or the percent of a product that failed, you will get three different estimates. These sample to sample differences are sampling variation. The size of this variation is an indication of the precision of an estimate. Precision is usually represented by the size of a confidence interval around an estimate. For example, a report may indicate that the percent of a product that failed is 15 percent with a confidence interval of plus or minus 4 percent at the 95 percent confidence level. This is saying that I am 95 percent confident that the true value of the population is within the range of 11 percent to 19 percent.
Sample size is important because the width of the confidence interval is affected by the size of the sample. Larger sample sizes typically will have narrower confidence intervals and therefore tend to be more precise. Small sample sizes may generate large confidence intervals and raise questions about whether the estimate is reliable or useful as evidence. For example, one court found that the sample size in a study of the risk of cancer from breast implants with a particular type of coating was too small and resulted in a very wide confidence interval that rendered the results of the study meaningless. The court wrote: "Based on the very limited sample of individuals with PUF-coated implants… the relative risk of PUFcovered implants was 1.99 with a margin of error between 0.5 and 8.0 at the 95 percent confidence level… This huge margin of error associated with the PUF-specific data (ranging from a potential finding that implants make a woman 50 percent less likely to develop breast cancer to a potential finding that they make her 800 percent more likely to develop breast cancer) render those findings meaningless for purposes of proving or disproving general causation in a court of law" (In re Silicone Gel Breast Implants Products Liability Litigation, 318 F. Supp.2d 879 (C.D.Cal. 2004); emphasis in the original).
While it is generally true that larger sample sizes result in a narrower confidence interval and therefore greater precision for an extrapolation, this relationship has diminishing returns. For example, Figure 1 shows the size of the confidence interval (on the vertical Y axis) around an estimate of 50 percent of a product failing (or of consumers with a particular opinion) for different sample sizes (along the horizontal X axis) using a 95 percent confidence level. With a small sample of 50 the confidence interval is plus or minus 14 percent meaning we are 95 percent confident the true value is between 36 percent and 64 percent. This estimate is not very precise. For a sample size of 500, this confidence interval is plus or minus approximately 4.4 percent. So the confidence interval is substantially narrower. Thus the larger sample size substantially improves the precision. However, if the sample size is increased to 1,000, the confidence interval only decreases to plus or minus 3.1 percent. So there are diminishing returns in terms of increased precision by increasing the sample size beyond a certain point. This relationship between sample size and precision is important since larger samples are more costly and time consuming to survey and analyse. It is often possible to achieve acceptable precision without needing to collect data from very large samples.
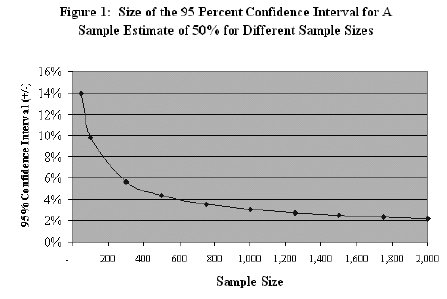
B. Questionnaire design and survey implementation Issues
The value of surveys in product liability cases also depends on the questions that are asked and the methods used to both ask and collect answers to the questions. For surveys to offer data that are probative to the case and reliable, the survey must be well designed and carefully executed. The questions must be clear, unbiased and not leading, and the interviewing or data collection procedures need to be carried out by qualified, professional staff.
In constructing questions for a survey, there are several important design considerations. First, it is important to have a clear understanding of the issues and facts of the case and how a particular question or question sequence will address those issues or facts. Second, questions should be unambiguous. Third, questions should not be written, presented or asked in a style that is leading i.e. poses the risk of potentially biased responses.
Survey questions for use in litigation need to be worded neutrally and should not be leading. Any clear evidence that responses may be a result of suggestion from the form or wording of the questions rather than the respondents’ best estimate of their opinion will lead to significant challenges by opposing experts. This includes not only the questions themselves, but the survey introductions to frame the questions, any instructions used in the survey, any transitions between questions, the order of the questions, the response categories, and any information that is shown to respondents as part of the survey.
One way in which survey answers can be potentially biased is by having the associated attorney or client heavily involved in the composition of the questions. Diamond explained in the Reference Guide on Survey Research, "the attorney should have no part in carrying out the survey. However, some attorney involvement in the survey design is necessary to ensure that relevant questions are directed to a relevant population" (Federal Judicial Center, Reference Manual on Scientific Evidence, Reference Guide on Survey Research (2d ed.2000), 229-276 at 237-238). In Tunnell v. Ford Motor Co., Plaintiff’s attorney wrote an "Information Piece" that was included in the beginning of the questionnaire. Plaintiffs maintained the piece merely presented a short description of the safety features of automobiles that were at issue in the case. Defendants alleged that the information piece was "essentially Tunnell’s counsel’s opening argument" and biased respondents from the outset of the survey (Tunnell v. Ford Motor Co., 330 F. Supp.2d 707 (W.D.Va. 2004)).
It is also important to carefully construct the description of the purpose and sponsors of the study. The standard practice is to explain the purpose of the study in a neutral way and to avoid directly naming the sponsor if that knowledge will bias or change a respondent’s answers. This is referred to as keeping the respondents "blind" to the true purpose of the survey in order to avoid introducing some type of response bias. Telling the respondent that the survey is to be used for litigation is particularly likely to lead to lower response rates and strategic answers due to respondents concerns about being involved in litigation and is therefore typically avoided. However, survey researchers have an ethical obligation to either keep respondents identities confidential or to provide sufficient disclosure that they understand what may be required of them after a survey is complete.
In addition to the questions, the survey implementation and the associated quality control procedures must be professionally done and meet standard industry practice. Sample surveys can be conducted in a variety of modes including telephone, selfadministered mail, in-person interviews, mall-intercept interviews, internet surveys and multi-mode combinations of these techniques. Each of these has strengths and weaknesses that will affect the choice for a particular case. Whatever the choice, the procedures need to be completed by qualified staff or interviewers with training in generally accepted data collection processes. Just as care must be used in designing questions to avoid bias, it is also important that the interviewers themselves do not introduce bias. If interviewers are being used to collect the data, one procedure that is used to minimise potential bias is also having the interviewers be "blind" to the purpose of the study. When both respondents and interviewers are blind to the purpose of the study then the study is referred to as "double-blind."
Finally, in statistical surveys where people are involved in either answering questions or in allowing access to the products or locations to be tested, the response rate to the survey will not typically be 100 percent. There are almost always some parts of the initial sample that cannot be contacted, that refuse to participate, or for some other reason fail to complete the data collection process.
To the extent that responses are not received from 100 percent of the sample, then questions arise about using the sample to provide a reliable estimate for the population. This concern results from the fact that if the sampled people for whom data are not received are different from those that responded in some important way, then the estimates from the sample will be potentially biased. This is called "nonresponse bias." A key consideration in evaluating a survey for use in product liability litigation is whether any nonresponse bias was introduced as a result of the procedures used to collect the data and the associated response rate. A survey report would generally explain what procedures were used to assess the likelihood of a nonresponse bias. This usually involves sampling non-respondents and comparing them with respondents on variables for which data is available either from other records or which can be appended from other data sources such as secondary data from demographic data companies.
C. Analysis and reporting issues
The use of sample survey data in product liability litigation also requires that the data gathered were accurately reported. Generally, sample surveys conducted by a professional survey firm will be subject to some degree of validation. This may involve having a separate firm call back survey respondents to confirm that they completed the survey or it may involve checking the survey responses against some available secondary data that could confirm responses to some questions. In addition, there will generally be checks on the internal consistency of responses within the survey.
Finally, the sample survey data and any associated measures with physical conditions need to be analysed in accordance with accepted statistical methods. There are a wide range of statistical methods that may be employed in analysing this type of data that qualify as "accepted statistical methods." Any guidelines set forth here must necessarily be broad. However, there are a few areas that can be identified for scrutiny in assessing the analysis of a sample survey in product liability cases. These include:
- Data Weighting . When a simple random sample is used, the estimate that is extrapolated to the population does not usually require weighted data. However, in many circumstances the population is first broken down into different subgroups (such as different cities, different manufacturing runs, different customer groups, or different demographic groups) and then random samples are drawn from the subgroups. If this data is then all combined into one sample for analysis, the data need to be weighted so that estimates from each subgroup have the proper proportional impact on the combined estimate. Improperly weighted data will affect the accuracy of any extrapolation to the population.
- Passage of time . Many product liability cases involve products that were produced and distributed through a period of years. There may have been changes in the manufacturing process or the handling process during this period of time. The sample data should have been collected to accurately reflect various periods of time and the analysis needs to address changes in the results over time. One criticism that was raised of the data collected in Perez v. John Deere Const. Equipment Co. was that it was not clear if the usage information collected on the cotton picking machines reflected information from machines over their entire 20- year useful life or only done with initial sales in the very beginning of the model’s useful life (Perez v. John Deere Const. Equip. Co., * WL 1529762 (Cal. Ct. App. 2005)).
- Geographical/Cultural differences . Many products are distributed and used over a wide geography. Differences across geography include such things as climate, environmental conditions, social differences in the use of products, purchase behaviors, use of communications channels and demographic profiles. Often these background factors will account for important aspects of the consumers’ understanding of and use of the products or the nature of their harm or damages. Yet frequently sample surveys will be conducted in limited geographical areas and then extrapolated to larger geographical areas without regard (or controls) for possible differences. The surveys that were relied upon in Muise v. GPU, Inc. were heavily criticised because they had been conducted on respondents who did not live in the geographic area affected by the power outage at issue. In addition the surveys were in reference to outages during different seasons than the outage at issue and did not address the occurrence of an outage on a holiday as was the case with the outage at issue (Muise v. GPU, Inc., 851 A.2d 799 (N.J. Super. A.D. 2004)).
- Use of control groups . In many product liability claims, causation is a key factor. In the analysis, an expert may attempt to establish causation by simply showing that the purported cause is present and the purported damage is present, therefore one caused the other. However, the inference of causality requires also demonstrating that when the purported cause is absent that the same damage does not occur and that the damage does not exist in the presence of other causes. If you study a sample of older green cars and find that they all have rust, it would be incorrect to conclude that green paint causes rust. You would have to study red cars and blue cars as well. Sorting out causality generally requires the use of appropriately designed control groups. Many product liability cases improperly lack an appropriate control group to determine whether the claimed damage is simply associated with the presence of the purported cause or whether there is sufficient reason to infer that the purported cause is in fact responsible for the damage.
V. Conclusions
Sample surveys are a useful tool to address a wide range of issues in product liability litigation and forecasting. This can include more traditional views of surveys measuring consumer "expectations," but also the use of surveys to understand purchase processes, estimate failure rates, evaluate economic choices under "but-for" conditions, and address issues in class certification. The value of sample surveys as used in litigation derives primarily from economies associated with studying random samples of people or products that can be extrapolated to the relevant population and the ability to systematically ask the same questions of the relevant population on issues that are probative to the case. These two advantages of sample surveys overcome the limitations of reliance on anecdotes and convenience samples when extrapolating or making inferences about the causes and consequences of product liability, particularly where large populations of a product, product purchasers, product users, or those exposed to a product are involved.
While a valuable tool, the uses of sample surveys are subject to many methodological issues that must be adequately addressed to ensure that the results are both reliable and likely to survive challenge. While each case will be different, the use of random sampling, the careful design and pretesting of questions and responses, and the application of analytical techniques that control for the many sources of variation in survey responses can help assure the successful use of sample surveys.
Acknowledgement
Paul Hinton and Frances Barlas are contributing authors to this article.
The content of this article is intended to provide a general guide to the subject matter. Specialist advice should be sought about your specific circumstances.